In today’s fast-paced customer service landscape, it’s not enough for companies to know what customers are saying—they need to understand how they’re feeling, too. This is where AI-driven sentiment analysis comes into play, providing crucial insights into customer emotions, attitudes, and satisfaction levels. As part of the latest customer service strategies, sentiment analysis enables companies to craft more personalized responses, anticipate customer needs, and create positive interactions. Let’s dive into how sentiment analysis works, its advantages in customer service, and the challenges and future possibilities of this powerful AI tool.
Table Of Contents
- 1 What is Sentiment Analysis in AI?
- 2 Key Benefits of Sentiment Analysis in Customer Service
- 2.0.1 1. Proactive Issue Resolution
- 2.0.2 2.More Personalized Customer Interactions
- 2.0.3 3.Efficient Inquiry Routing
- 2.0.4 4. Enhanced Employee Training and Feedback
- 2.0.5 5.Data-Driven Business Insights
- 2.0.6 Challenges of Implementing Sentiment Analysis in Customer Service
- 2.0.7 1.Accuracy and Context Recognition
- 2.0.8 2.Multilingual and Cultural Sensitivity
- 2.0.9 3.Data Privacy and Ethical Considerations
- 2.0.10 4.Continuous Monitoring and Updates
- 2.0.11 The Future of Sentiment Analysis in Customer Service
- 2.0.12 1.Emotion Detection and Enhanced Empathy
- 2.0.13 2.Multimodal Sentiment Analysis
- 2.0.14 3.Predictive Analytics and Customer Retention
- 2.0.15 4.Integration with Voice-Activated Customer Service
- 3 Conclusion
What is Sentiment Analysis in AI?
Sentiment analysis, often called “opinion mining,” is an AI-driven process that decodes the emotional tone of customer messages. By analyzing text, voice, and potentially even images, sentiment analysis gauges whether a customer’s message is positive, negative, or neutral. This understanding allows companies to respond in ways that feel more personalized and in tune with customers’ needs.
The technology behind sentiment analysis leverages Natural Language Processing (NLP) and machine learning. NLP enables AI to recognize language patterns, identifying words and phrases linked to certain emotions, while machine learning models are trained to classify these emotions with accuracy based on historical data. For example, phrases like “I’m delighted with this service” are flagged as positive, while “This is unacceptable” is marked as negative.
By processing large amounts of customer interactions, sentiment analysis provides real-time insights, helping customer service teams track customer sentiment and ensure issues are managed effectively
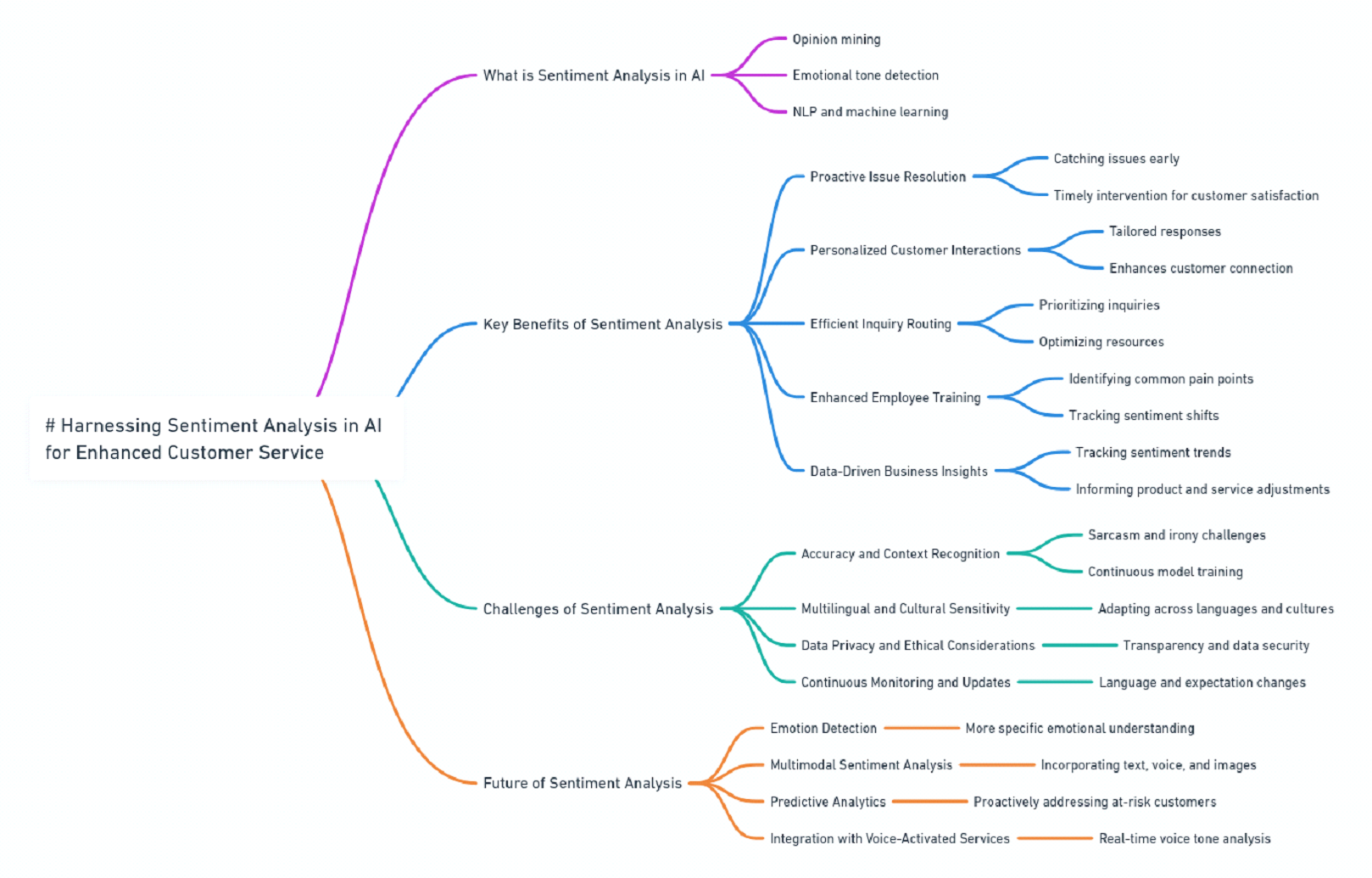
Key Benefits of Sentiment Analysis in Customer Service
1. Proactive Issue Resolution
Sentiment analysis helps teams catch potential issues early. If an AI system picks up repeated expressions of frustration, it can alert a human agent to step in. This proactive approach prevents small frustrations from growing and shows customers that their concerns are being addressed quickly. Such timely intervention builds customer satisfaction and loyalty by showing genuine care..
2.More Personalized Customer Interactions
Sentiment analysis allows AI to tailor responses based on a customer’s tone, moving beyond generic replies. For instance, if frustration is detected, the AI might suggest a more empathetic, apologetic response. A positive message, on the other hand, could be met with appreciation or encouragement. By adding a personal touch, sentiment analysis ensures customers feel understood, even in high-volume interactions, helping to maintain a strong connection.
3.Efficient Inquiry Routing
Sentiment analysis aids in routing customer inquiries to the appropriate channels based on urgency. When a highly negative message is flagged, it can be prioritized and sent to a skilled agent who can handle complex issues with care. Neutral or positive inquiries might be addressed by automated responses or junior agents. This strategic routing allows teams to manage resources effectively and respond to high-priority cases promptly.
4. Enhanced Employee Training and Feedback
Sentiment analysis provides data that’s useful for improving training. Patterns in customer sentiment can reveal common pain points, areas that need clarification, or opportunities for enhancing customer service. For example, if multiple customers express confusion about a product, that insight can inform additional training for support agents.
Moreover, sentiment analysis serves as a feedback tool, tracking shifts in sentiment before and after interactions. Managers can use this data to assess agent performance, leading to more targeted training and skill development that ultimately elevates service quality.
5.Data-Driven Business Insights
Sentiment analysis also offers broader insights into customer preferences and satisfaction. By examining sentiment trends over time, companies can identify popular products, frequent complaints, and shifts in customer satisfaction. These insights help guide business decisions, from product improvements to service policy adjustments. For example, a trend of negative sentiment around a product might prompt a quality review, while positive feedback on a new feature could shape future product developments.y.
Challenges of Implementing Sentiment Analysis in Customer Service
While sentiment analysis has clear advantages, it also presents some challenges:
1.Accuracy and Context Recognition
Language can be nuanced, and emotions aren’t always expressed clearly. Irony, sarcasm, and regional dialects can make it challenging for AI to interpret sentiments accurately. A phrase like “Just great!” could be genuine or sarcastic, depending on the context. To improve accuracy, sentiment analysis models need to be trained continuously on diverse language patterns, though achieving total accuracy remains difficult, especially in informal or slang-heavy language
2.Multilingual and Cultural Sensitivity
For companies with a global presence, customer service must operate across languages and cultures. Sentiment analysis tools need multilingual capabilities to ensure accuracy for all customers. Cultural differences in expressing emotions also mean that AI models need extensive training to adapt across regions and avoid misinterpretation.
3.Data Privacy and Ethical Considerations
Since sentiment analysis relies on customer data, privacy and ethics are crucial. Companies must be transparent about how they collect and use customer data, following regulations like GDPR to maintain customer trust. Customers may be uncomfortable knowing that AI is analyzing their emotions, so businesses should clearly explain their data use policies and prioritize data security.
4.Continuous Monitoring and Updates
Sentiment analysis tools require ongoing adjustments to stay relevant. As language evolves, so must AI models to ensure they continue to understand current trends and customer expectations. Regular updates and real-time monitoring are essential to maintain accuracy, especially in applications that directly affect customer interactions.
The Future of Sentiment Analysis in Customer Service
The potential for sentiment analysis in customer service is huge, with advances in AI and machine learning promising even greater capabilities. Here’s a look at what the future might hold:
1.Emotion Detection and Enhanced Empathy
Beyond identifying positive or negative tones, future sentiment analysis tools may detect more specific emotions like joy, anger, or surprise. With this deeper emotional understanding, AI could offer responses that are even more empathetic and suited to the customer’s needs, leading to customer experiences that feel uniquely supportive.
2.Multimodal Sentiment Analysis
Sentiment analysis may soon expand beyond text and voice, incorporating images and video to analyze customer emotions. This multimodal approach could enable customer service to interpret facial expressions or body language during video calls, providing a more comprehensive understanding of a customer’s emotional state. This could be especially useful in healthcare or finance, where a detailed grasp of emotions is key to building trust.
3.Predictive Analytics and Customer Retention
Sentiment analysis could combine with predictive analytics to help businesses identify customers at risk of leaving. By monitoring shifts in sentiment, companies could proactively reach out to at-risk customers, addressing issues before they lead to churn. This capability would be invaluable in customer retention strategies across sectors like telecommunications, where keeping customers loyal is crucial.
4.Integration with Voice-Activated Customer Service
As voice-activated assistants like Siri, Alexa, and Google Assistant become more common, sentiment analysis could soon play a role in voice-based customer service. AI-powered voice sentiment analysis could interpret not just the words spoken but also the tone, speed, and pitch, giving businesses real-time insight into how customers are feeling during live conversations.
Conclusion
Sentiment analysis in AI is transforming customer service, offering a new depth of insight into customer emotions, enhancing personalization, and supporting proactive responses. Though challenges like accuracy, cultural nuances, and privacy concerns remain, sentiment analysis is steadily advancing toward greater accuracy and effectiveness. For companies that embrace it, sentiment analysis offers a path to deeper customer relationships, higher satisfaction, and a customer service experience that feels genuinely empathetic.
As companies continue to invest in this technology, sentiment analysis is setting the stage for a new era of customer service where understanding emotions and delivering personalized interactions become as important as solving issues. This evolution promises to not only enhance customer loyalty but to reshape how brands connect with and support customers in ways that are impactful and meaningful.